There was a time when adding a simple "Dear X" to a canned email was considered personalization. Today, artificial intelligence (AI) can possibly predict your next purchase in real time and display only the most relevant products when you visit a website.
This shift has been underscored by significant investments in data architecture, analytics, machine learning (ML) models, and, most importantly, buy-in from leadership. As generative AI (GenAI) reshapes the landscape, we now stand on the cusp of hyperpersonalization at scale. At the core of this transformation is the organization’s ability to effectively harness and utilize data.
The Hyperpersonalization Value Chain
Data is the lifeblood of hyperpersonalization as it fuels ML models and drives intelligent recommendations. Yet, in many enterprises, it remains dark—unstructured or semi-structured and requires cleansing and transformation. Data comes in formats in real-time and in batches from diverse sources, such as legacy systems, new tools, and AI applications.
This is where data governance and master data management play a crucial role. To achieve hyperpersonalization, data must be transparent, complete, and free from bias. Once the data is standardized, it is used to build algorithms that provide actionable insights through predictions, recommendations, or simulations. However, merely generating insights is not enough. The next critical step is embedding these insights into operational workflows, processes, or applications centered on delivering superior user experiences.
From Segmentation to Hyperpersonalization
Looking back, personalization started with simple customer segmentation. Marketers grouped customers into broad segments based on their basic purchase history or manually provided data.
A confluence of factors led to the next phase of personalization—data generation along the customer journey exploded, real-time data capture improved, and computing costs decreased. These changes made it possible to analyze and predict customer behavior in ways that were more valuable than ever before.
Retail and banking were early adopters of this evolution. For example, Amazon's predictive algorithms or Starbucks' personalized campaigns. Soon after, healthcare, life sciences, and B2B sectors followed.
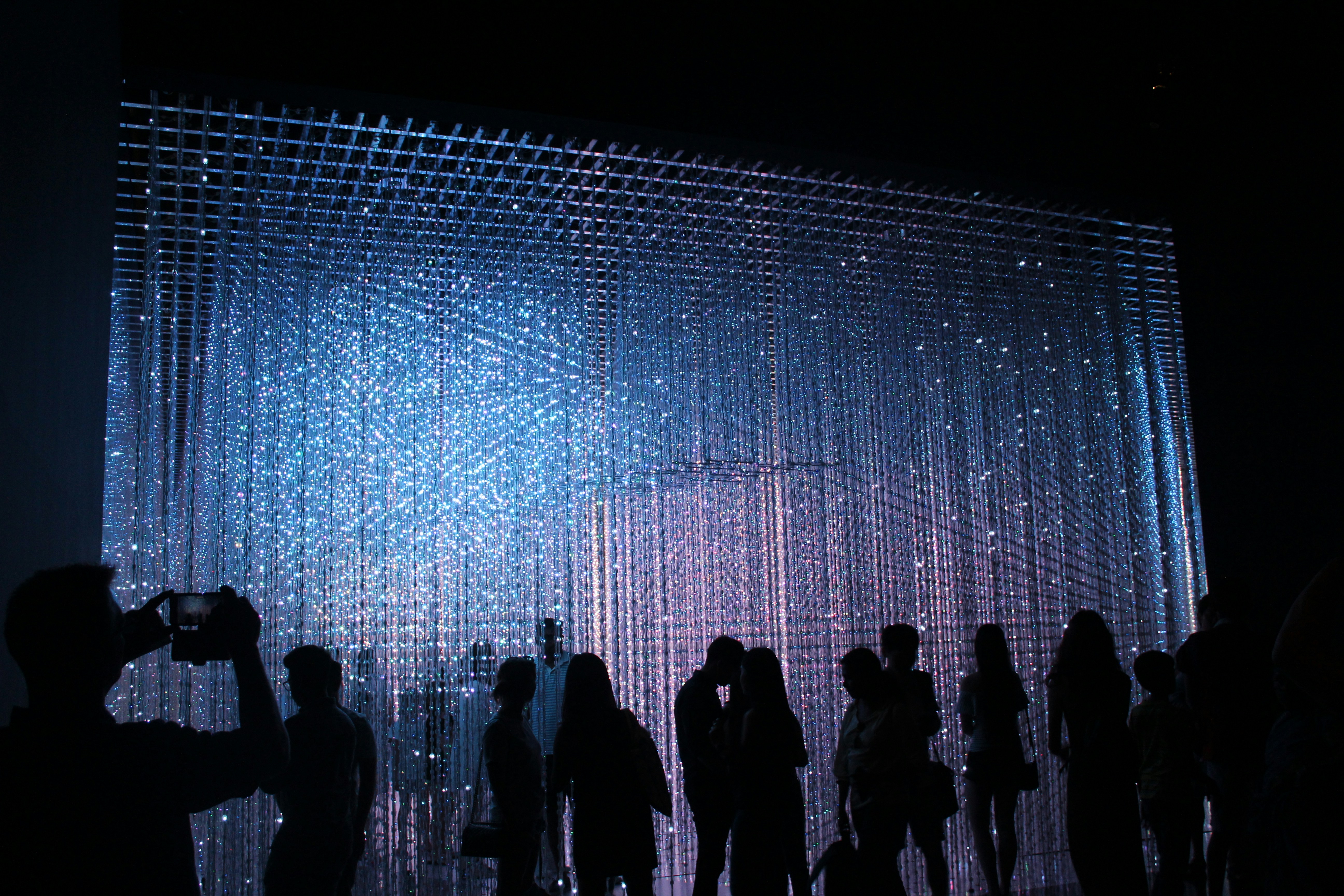
Real-world Applications of Hyperpersonalization
With falling compute costs, emerging data sources, and real-time customer insights, hyperpersonalization is the next frontier. Media companies such as Netflix (with hyperpersonalized movie categories), Spotify (with custom playlists), and e-commerce leaders like Amazon (leveraging collaborative filtering) are great examples of hyperpersonalization in action.
However, it only works when we can connect customers across all touchpoints of their journey, in real time, while delivering the right messages through the right channels.
For example, we recently helped a global gaming and entertainment company migrate its on-premises big data to Azure, a move that enabled scalability. By transferring legacy data, using tools like Databricks and Azure SQL, and building Power BI dashboards, we empowered stakeholders with real-time insights, enhancing decision-making and personalization.
On the other hand, for a North American media company, we recently automated a process to understand the number of viewers per household, providing invaluable insights to the ad-sales team. This required merging diverse datasets—such as Nielsen and in-house measurement data—within an AWS instance and creating rule-based engines for the targeted segmentation, a leap from traditional data segmentation practices.
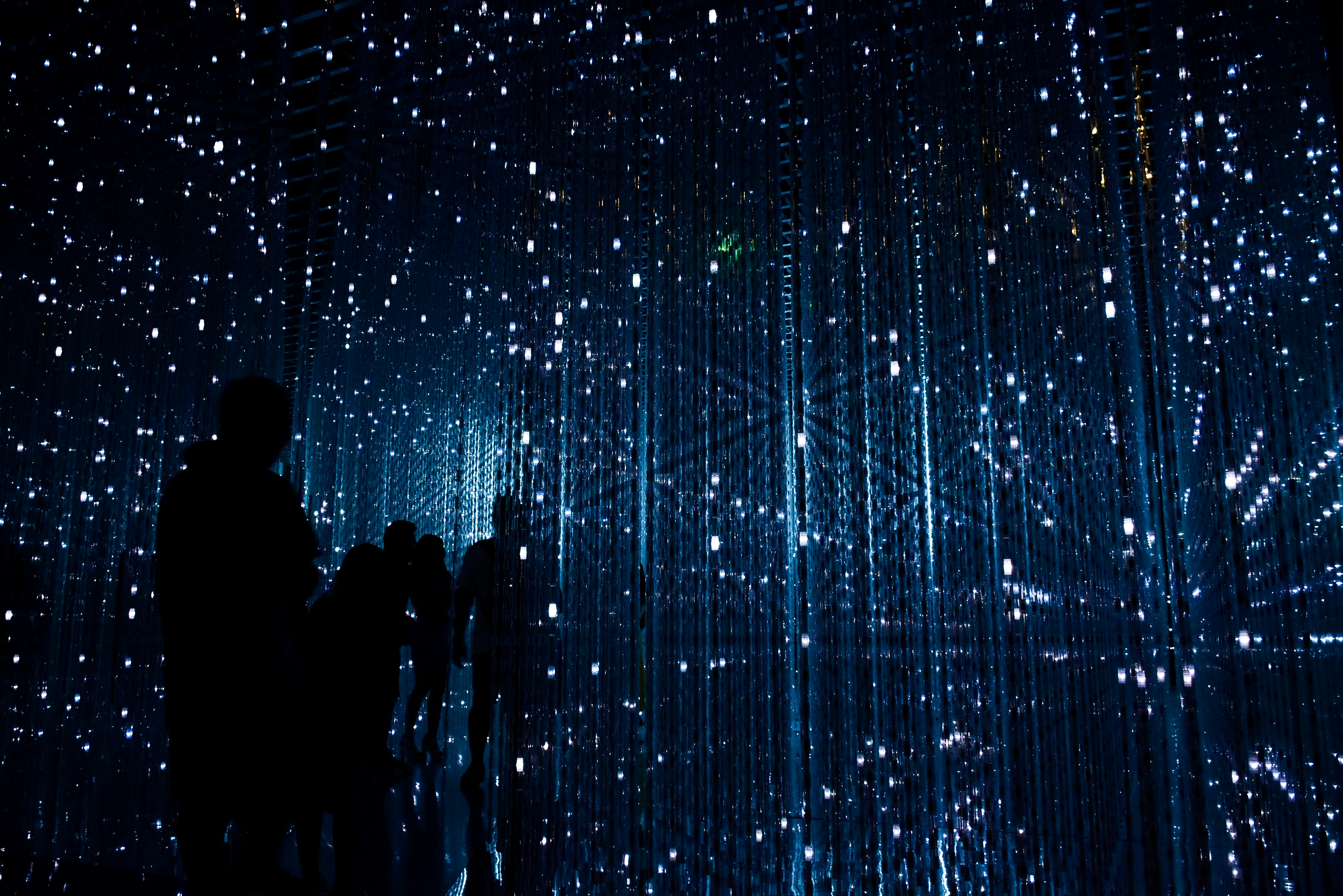
GenAI and the Future of Hyperpersonalization
With the democratization of AI, we now have unprecedented opportunities. Imagine a GenAI system that not just recommends products based on browsing history or purchase trends but crafts an entire outfit from eco-friendly brands based on your style preferences. Or envision predictive inventory management that uses individual customer data alongside broader market trends to predict purchases, ensuring that only relevant products are stocked, reducing the need for future clearance sales.
As an example, we recently partnered with a media client on a GenAI-based budget allocation project for optimized content investment. By integrating third-party market research data and internal APIs, we leveraged Generative Adversarial Networks (GANs) for unsupervised learning. After optimizing the models, we maximized the client's ROI through a tailored content classification strategy, providing simulations for different budget allocation scenarios.
The possibilities are endless. However, no algorithm can function effectively without clean, structured, and accurate data. Hyperpersonalization at scale depends on it.