As companies seek to unlock the full potential of artificial intelligence (AI), large language models (LLMs) have emerged as transformative tools. Yet, while LLMs are undeniably powerful, their performance can be inconsistent, especially when working with complex or nuanced information. This is where knowledge graphs come into play. By organizing data in a more meaningful way, knowledge graphs can elevate the capabilities of LLMs, enabling them to deliver smarter, more efficient, and more contextually accurate responses.
Gartner's February 2024 report on emerging technologies identified Generative AI (GenAI) and Knowledge Graphs as critical innovations for companies to invest in over the next year. But how exactly can knowledge graphs enhance LLM performance, and what tangible benefits do they bring to the table for forward-thinking businesses? Let us start by decoding knowledge graphs.
What is a Knowledge Graph?
Imagine data represented as a vast network of relationships. A knowledge graph maps connections between entities—people, products, places, and concepts—creating a web of context that LLMs can draw upon. Unlike traditional databases, which store data in isolated rows and columns, knowledge graphs capture not just the facts but the intricate relationships between them. This provides a more dynamic and intelligent framework for querying data.
By structuring data in this way, knowledge graphs offer a deeper semantic understanding. For example, instead of simply knowing that "John works for Company X," a knowledge graph reveals the broader context: John's role, the industry Company X operates in, and perhaps even the relationships between Company X and other organizations. This semantic depth allows knowledge graphs to deliver highly relevant insights that are often lost in conventional databases.
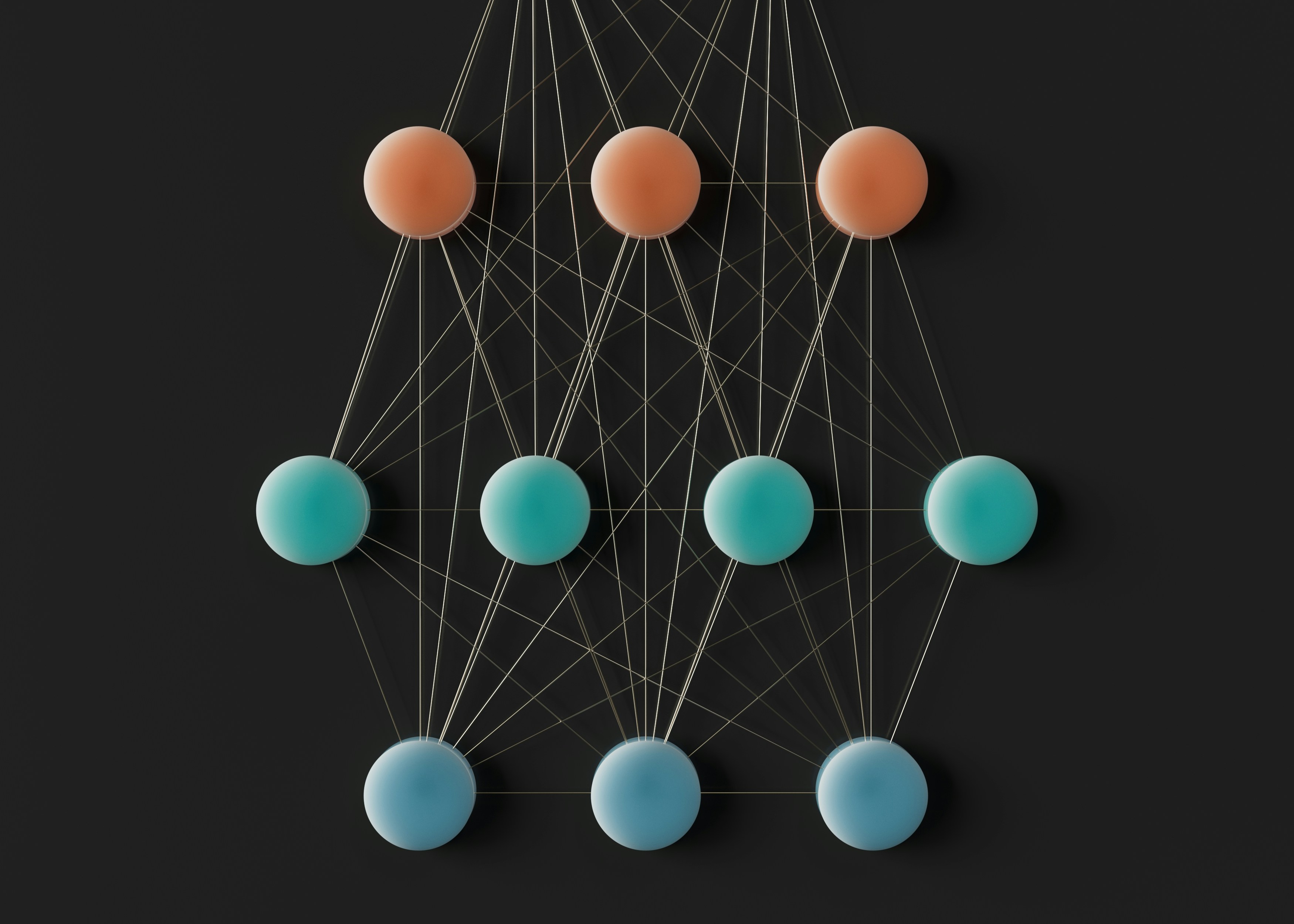
Three Approaches to Enhance LLMs with Knowledge Graphs
There are three primary ways companies can integrate knowledge graphs with their LLMs to drive superior performance:
-
Directly Combining Structured Data
One of the simplest methods involves feeding structured data, such as CSV or RDF files, directly into LLMs. This provides the model with additional data points to inform its output. Imagine an LLM writing a report on current market trends. By integrating an additional real-time financial dataset into its workflow, the LLM can generate far more accurate and up-to-date insights than if it were relying solely on pre-trained data.
-
Utilizing Raw Metadata and Graphs
Another approach involves augmenting LLMs with raw metadata—like timestamps, locations, or author names—paired with segments of a knowledge graph. This gives the model a richer understanding of context. For instance, in the healthcare sector, combining patient metadata with a biomedical knowledge graph allows the LLM to generate more tailored and precise case summaries, aiding in medical decision-making.
-
In-context Learning with Graph Embedding
The third, and perhaps most sophisticated, strategy is using knowledge graphs for in-context learning. This involves embedding relevant snippets of the knowledge graph directly into the LLM’s input prompts. By doing this, the LLM is guided to generate responses that align more closely with the structured data it has been provided. For example, in the case of an AI healthcare assistant, embedding parts of a diabetes knowledge graph in the prompts would enable the LLM to generate more accurate and medically sound information on symptoms and treatments.
Knowledge Graphs and Cost Efficiency
Apart from improving the accuracy and power of contextual responses, knowledge graphs are also making a significant impact on the cost efficiency of running LLMs. The high computational expense of generating responses—estimated at around $0.36 per query—can quickly add up for businesses, particularly when LLMs are required to answer repetitive or similar questions. Here, knowledge graphs can reduce costs by storing historical responses. Instead of regenerating the same answers repeatedly, the LLM can retrieve previous outputs directly from the graph, saving time and resources. Moreover, by converting unstructured data (such as documents or web pages) into a knowledge graph, companies can enhance information retrieval, further reducing inference costs and improving accuracy.
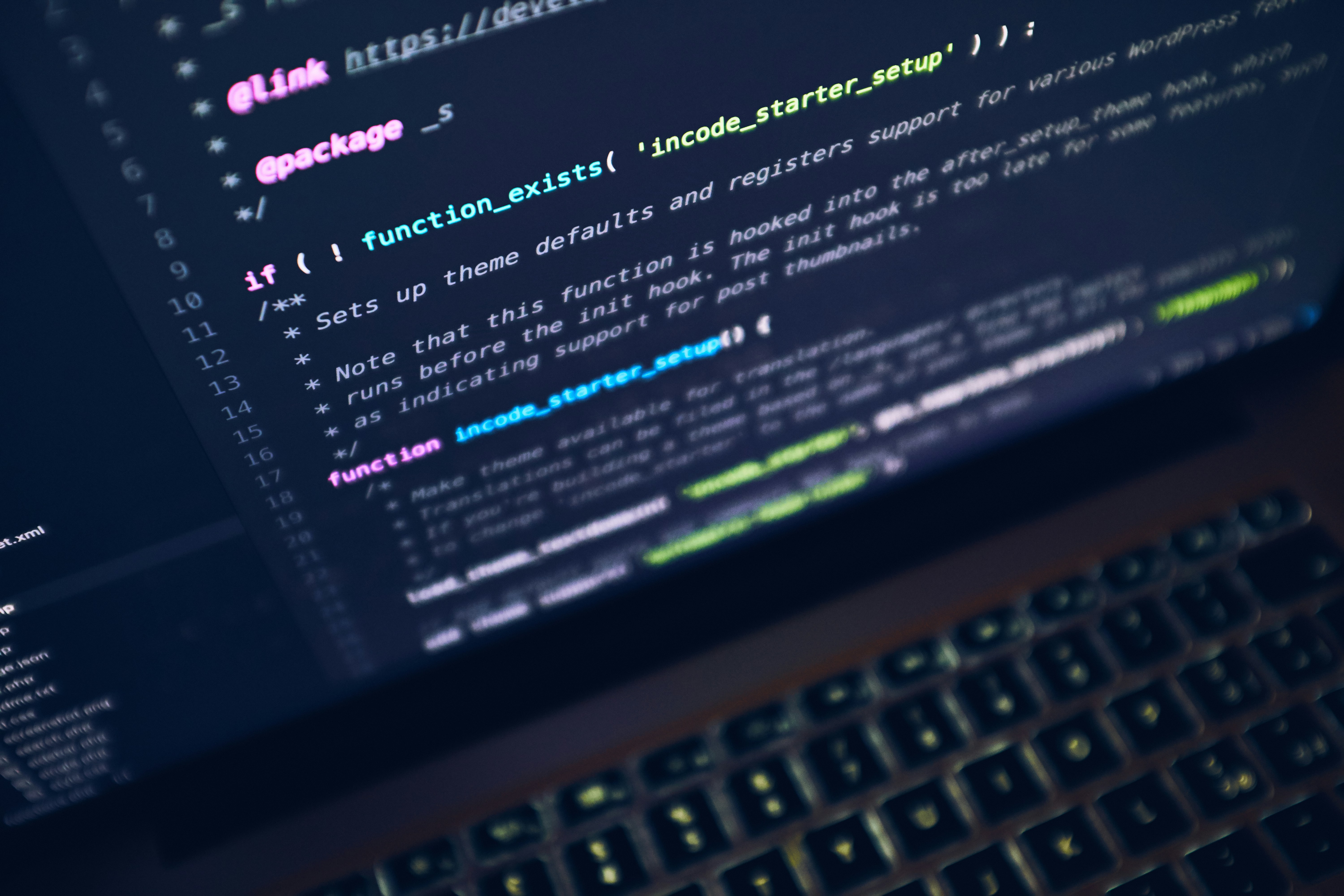
The Big Convergence: Knowledge Graphs and Data Management
As the volume of data grows exponentially, companies are grappling with how to manage both structured and unstructured information. Hybrid cloud environments often exacerbate this challenge by spreading data across different locations and formats. Knowledge graphs present a compelling solution to this problem by creating a unified structure that allows LLMs to navigate and retrieve information efficiently. In fact, we are on the cusp of a Big Convergence in data and knowledge management, where structured and unstructured data no longer operate in silos. Knowledge graphs will play a pivotal role in this transformation, helping businesses organize their data more intelligently and ensuring that LLMs can access and utilize it effectively.
A Strategic Imperative for CXOs
For CXOs, the message is clear: The future of AI-driven business lies in the synergy between LLMs and knowledge graphs. Together, these technologies offer a pathway to smarter, more efficient operations—whether it is delivering hyper-personalized customer experiences, automating complex business processes, or enhancing decision-making with real-time insights. The companies that invest in this convergence today will be the ones leading the AI revolution tomorrow.